Help Center
Data Visualization techniques for Identifying patterns and Trends
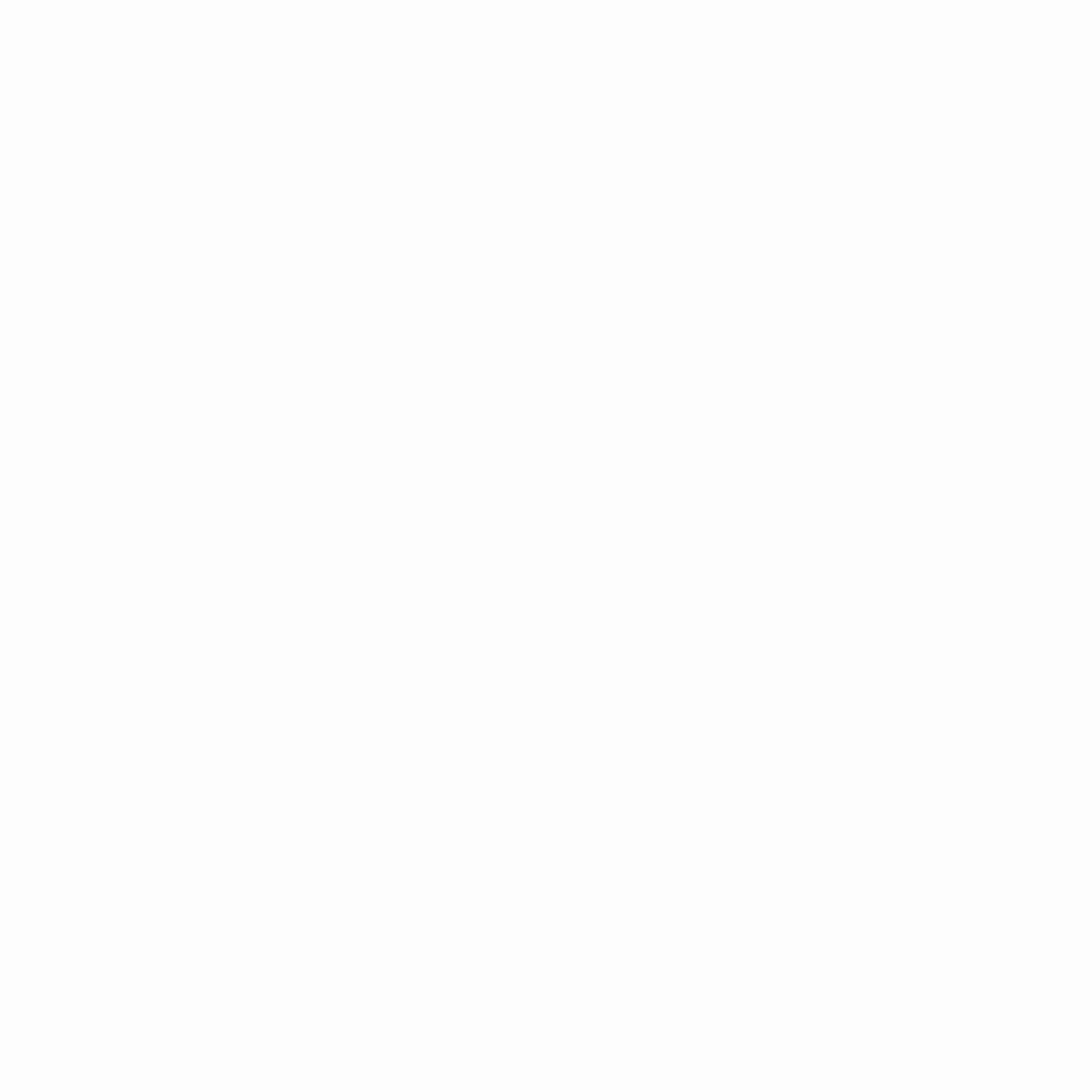
Data opens up remarkable opportunities if you understand what the data is trying to say. However, raw data is available in an unstructured, uncluttered way, which could be more useful. To extract information, data should be presented logically so that you can analyze the data correctly. That’s where data visualization comes into the picture. This article will help you learn about data visualization and different techniques to identify patterns and trends.
What is data visualization?
In layman’s terms, data visualization organizes the procured data as a graphical representation. This allows you to see and easily understand data trends, outlines, and patterns. But why is data visualization a must for any business?
Importance of data visualization
In modern days we generate 2.5 quintillion tons of data every day. Therefore it becomes difficult to determine what’s relevant or not when so much data is flying around every day.
With data visualization, one can visualize data and comprehend it faster. Whether a business or a career, data visualization is the most effective way to deliver data to familiar people. In the process, raw data is processed, modeled, and delivered so anyone can make data-driven decisions. Data visualization can be generated through different techniques, which are as follows
Different techniques for data visualization
There are three types of techniques under which different techniques can be used which are
- Univariate Analysis – In this single feature is used to analyze all the properties.
- Bivariate Analysis – Comparison of 2 features is known as Bivariate analysis
- Multivariate analysis – In this, we will be comparing more than 2 variables.
Now let us understand different techniques under data visualization
- Box Plot
The box plot is a standardized way of displaying the distribution of data. In this, the straight line at the minimum and maximum are called whiskers. You can identify the outliers by analyzing the points outside the whiskers. It can also help you know if your data is symmetrical and whether it is tightened in the group.
A box plot lets you know how the data values are spread out. Also, they take up less space which is helpful when comparing the distribution between many groups and datasets. Though some dataset requires more information than the measure of central tendency
2. Distribution Plot
We can use a distribution plot to determine the effect of a target variable (output) on an independent variable (input). It combines the probability density function and density in a single plot.
3. Violent Plot
The violent plot is a composite of a box plot in the center and a distribution plot on both sides of the data. This allows you to describe the dataset, such as whether it is multimodal or skewed, and so on.
4. Line Plot
The most common technique, a line plot, is used to know the dependency of one variable over another. It is represented in values on the series of data points connected with a straight line. The plot has various applications in many areas.
5. Bar Plot
Bar plot is widely used to compare the quantities of different categories or groups. Values in the categories are represented through bars, and they can be presented in horizontal or vertical form to analyze the data like sales figures, revenue from the product, number of visitors on a daily or weekly basis to a site, etc.
6. Scatter plot
It is one of the simplest plots to visualize data in machine learning or data science. It represents each point in the dataset that is present concerning any 2 or 3 features. Scatter points can be viewed in both 2D and 3D, through which we can find the Patterns and clusters of the data.
7: Line Charts
Line charts are one of the most common data visualization techniques, and they are useful for displaying trends over time or continuous data. Line charts are excellent for identifying patterns in data and visualizing changes in values over time.
8. Geographic Maps
Geographic maps are used to visualize data and help identify regional patterns or trends. Geographic maps are often used to visualize demographic, sales, or healthcare data.
9. Heat Maps
Heat maps are a graphical representation of data where values are represented by color. They help visualize and identify sets and identify patterns within the data. Heat maps are often used in finance, biology, and meteorology fields.
10. Bubble Charts
Bubble charts are similar to scatter plots but add a third dimension to the data. In a bubble chart, the size of the bubbles represents a third variable, and bubble charts help identify trends and correlations between three variables.
Conclusion
In conclusion, data visualization is an essential tool for identifying patterns and trends in data. Using these visualization techniques, we can turn complex data into meaningful insights that inform decision-making. Whether you are analyzing sales data, survey results, or healthcare data, there is a data visualization technique that can help you gain a deeper understanding of your data. We hope you have increased your knowledge about data visualization techniques from the above information. To learn more, visit Education Nest.