Help Center
The Data Science Boom and Future Trends To Consider
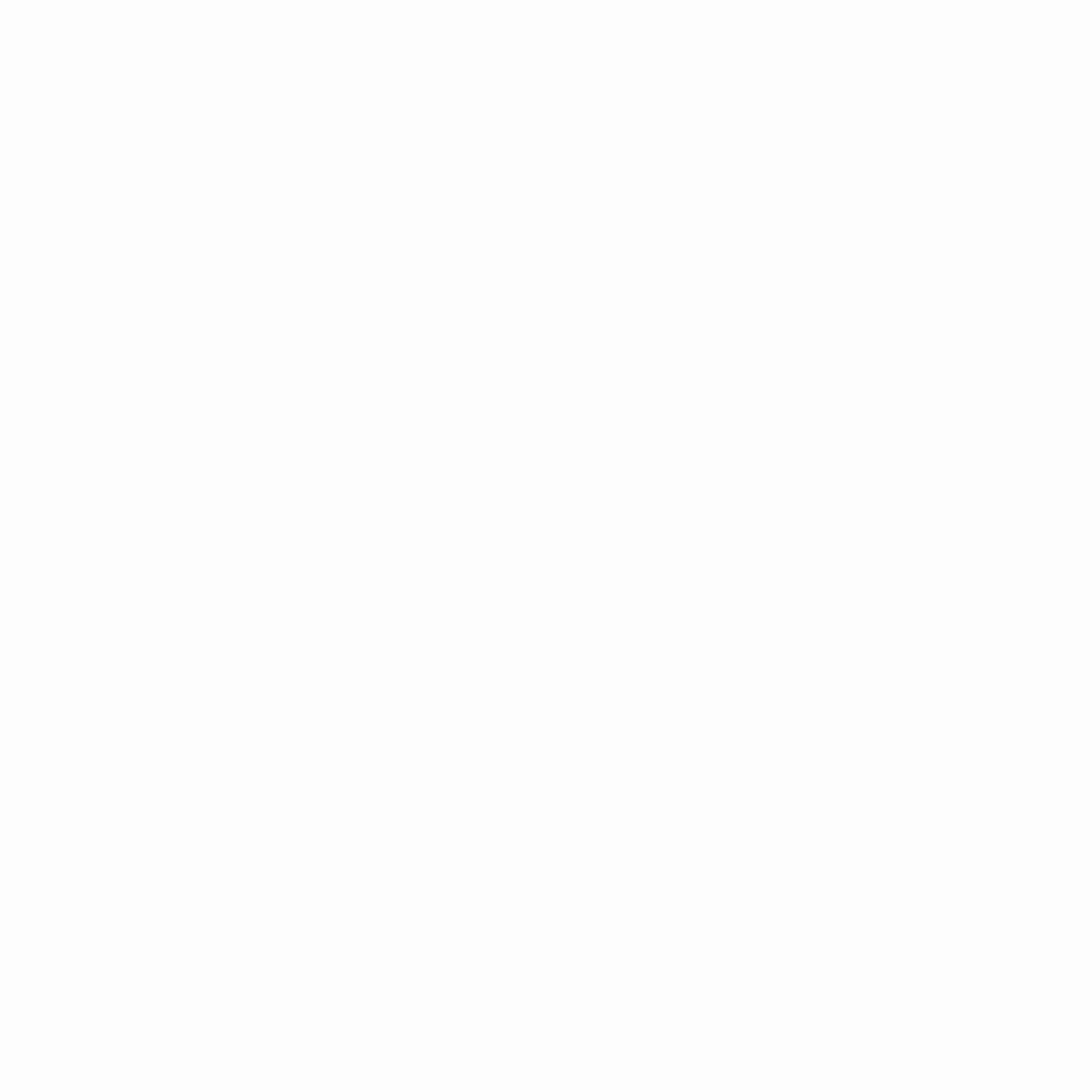
Data science combines math, statistics, specialized algorithms, sophisticated analytics, AI, and machine learning (ML) with highly efficient subject matter expertise that aims to discover valuable information in any corporation’s database. This information can be implemented to aid strategic planning and decision-making.
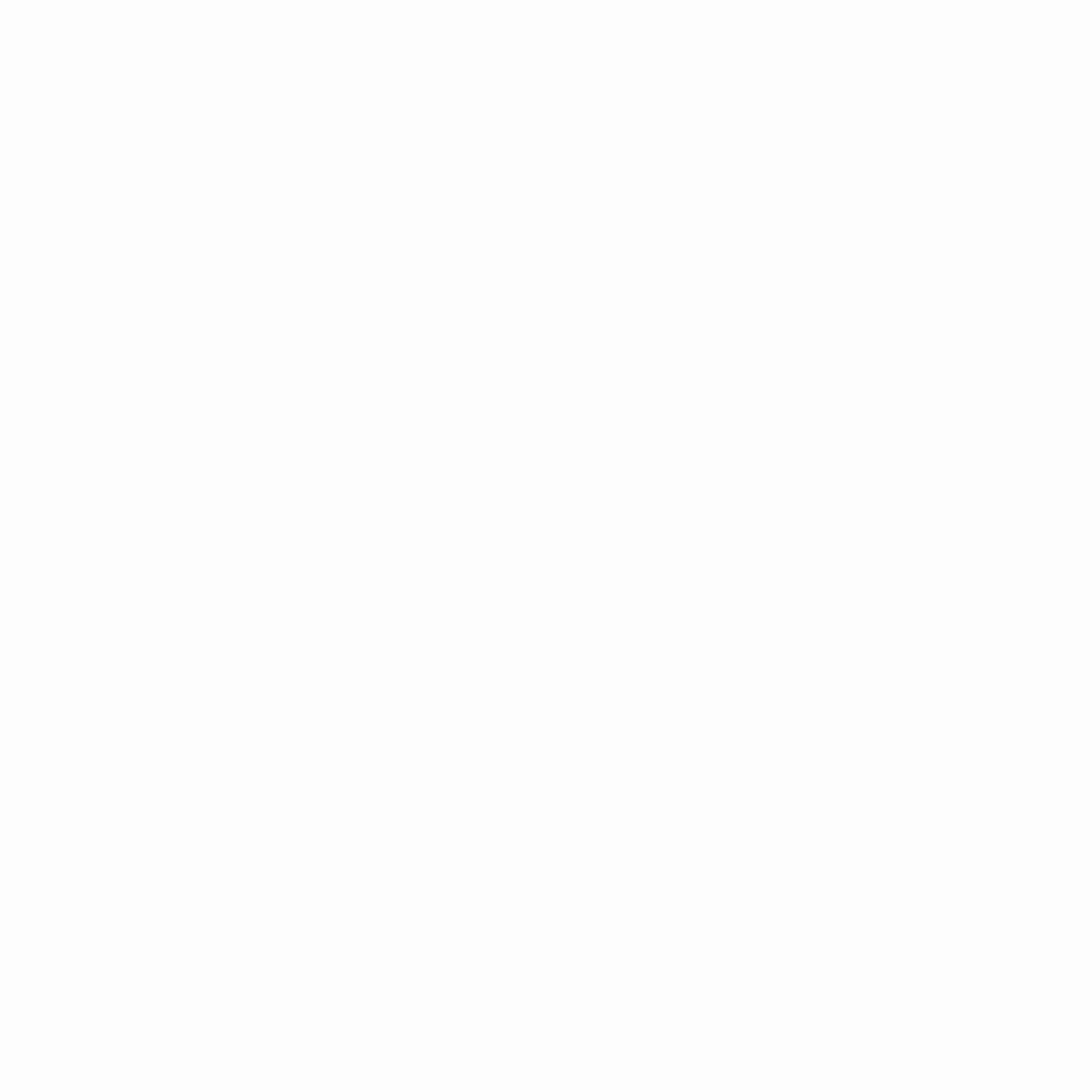
Let’s consider a practical example:
Credit risk assessment is a common application of data science in the financial industry. Let’s say, you walk into your nearest bank to apply for a lump-sum business loan. But you may end up becoming a defaulter, and the bank’s assets are lost. So how can a bank minimize the risk?
Stage 1:
Data collection: Data scientists gather huge amounts of data, including financial statements, credit history, employment history, and demographic data. This data builds your comprehensive profile.
Stage 2:
Data pre-processing: The collected data is usually unstructured and needs sorting and cleaning before it is of any significance. Data scientists use data wrangling, data cleaning, and data transformation techniques to polish the data for analysis.
Stage 3:
Feature engineering: Data scientists extract features from the data that capture vital aspects of your financial history and demographic characteristics. For example, they may extract features, such as income, debt-to-income ratio, credit score, age, and employment history.
Stage 4:
Machine learning: Data scientists use ML algorithms to analyze the data and predict the likelihood of you defaulting on a loan. Some common techniques used in credit risk assessment include logistic regression, decision trees, and random forests.
Stage 5:
Evaluation: Data scientists analyze the performance of the credit risk assessment model by comparing the predicted default rates to the actual default rates. Accuracy, precision, and recall metrics are used to evaluate the performance of the model and identify areas for improvement.
Using data science, financial institutions can make more accurate and informed lending decisions, which can help them minimize losses and reduce NPAs. At the same time, you can benefit from more personalized and fair lending decisions based on your personal financial history and demographic characteristics.
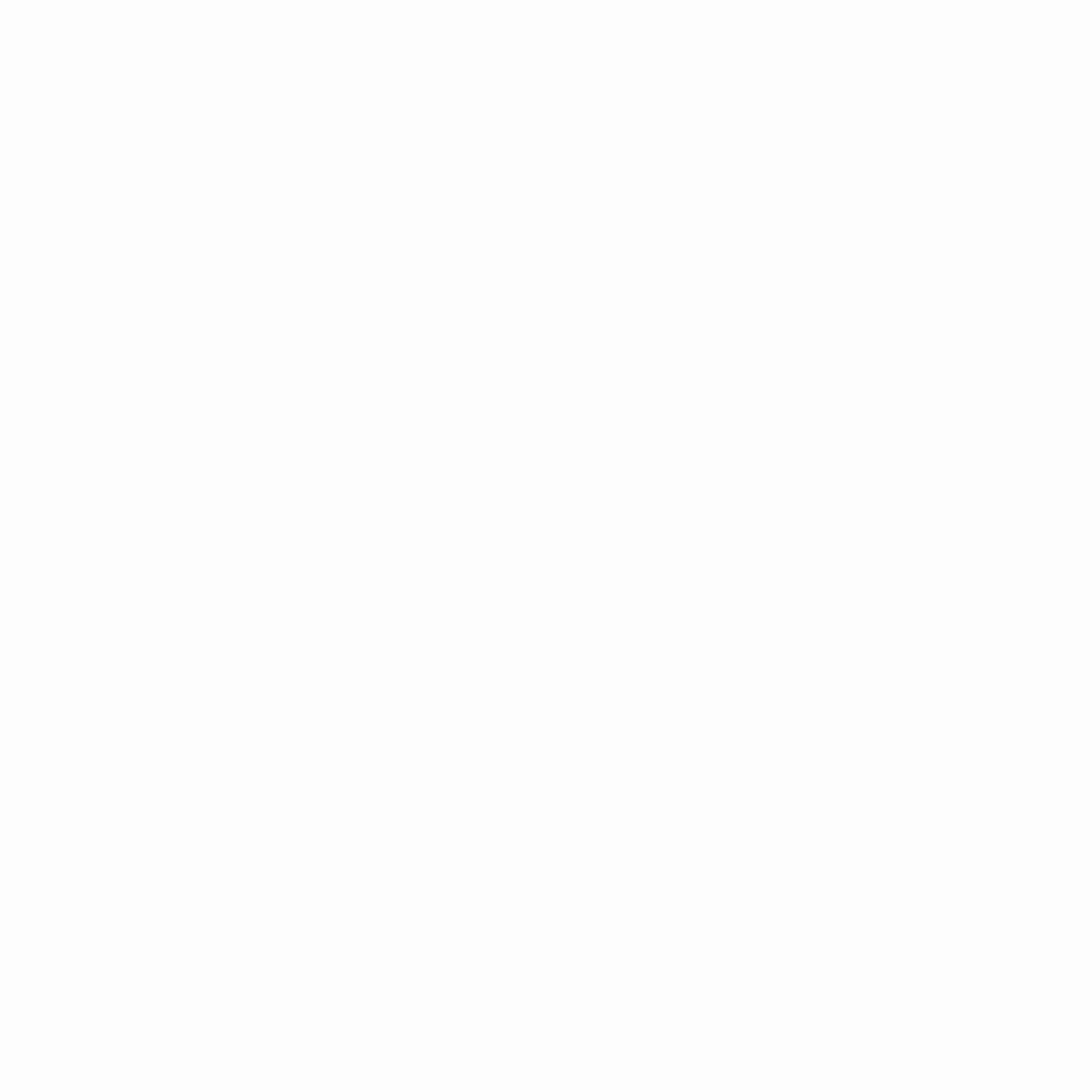
Future Trends in Data Science Applications:
1. Small Data and TinyML
Small data processes data rapidly and effectively in situations where bandwidth and processing time are limited. TinyML algorithms take up the least amount of memory and use the least amount of power. These devices include wearable, automobile, agricultural, and industrial machinery.
2. Data-Driven Consumer Experience:
As customers’ demands increase, businesses are looking for more sophisticated data science solutions to provide them with personalized experiences. Some examples are personalized recommendations, tailored content, and targeted ads.
3. Cloud computing:
Approximately 67% of businesses have shifted their big data to cloud systems as of 2022. Businesses are actively using Cloud services for data storage, processing, and delivery.
4. Auto ML:
AutoML is a trend that is “democratizing” ML in addition to “democratizing” data science. AutoML allows data scientists to automate the entire process of building machine learning models, which leads to faster model creation and deployment.
5. Artificial Intelligence as Service (AIasS):
Deep learning and AI-driven solutions have become increasingly popular in the last few years and have become a major focus for many data science professionals.
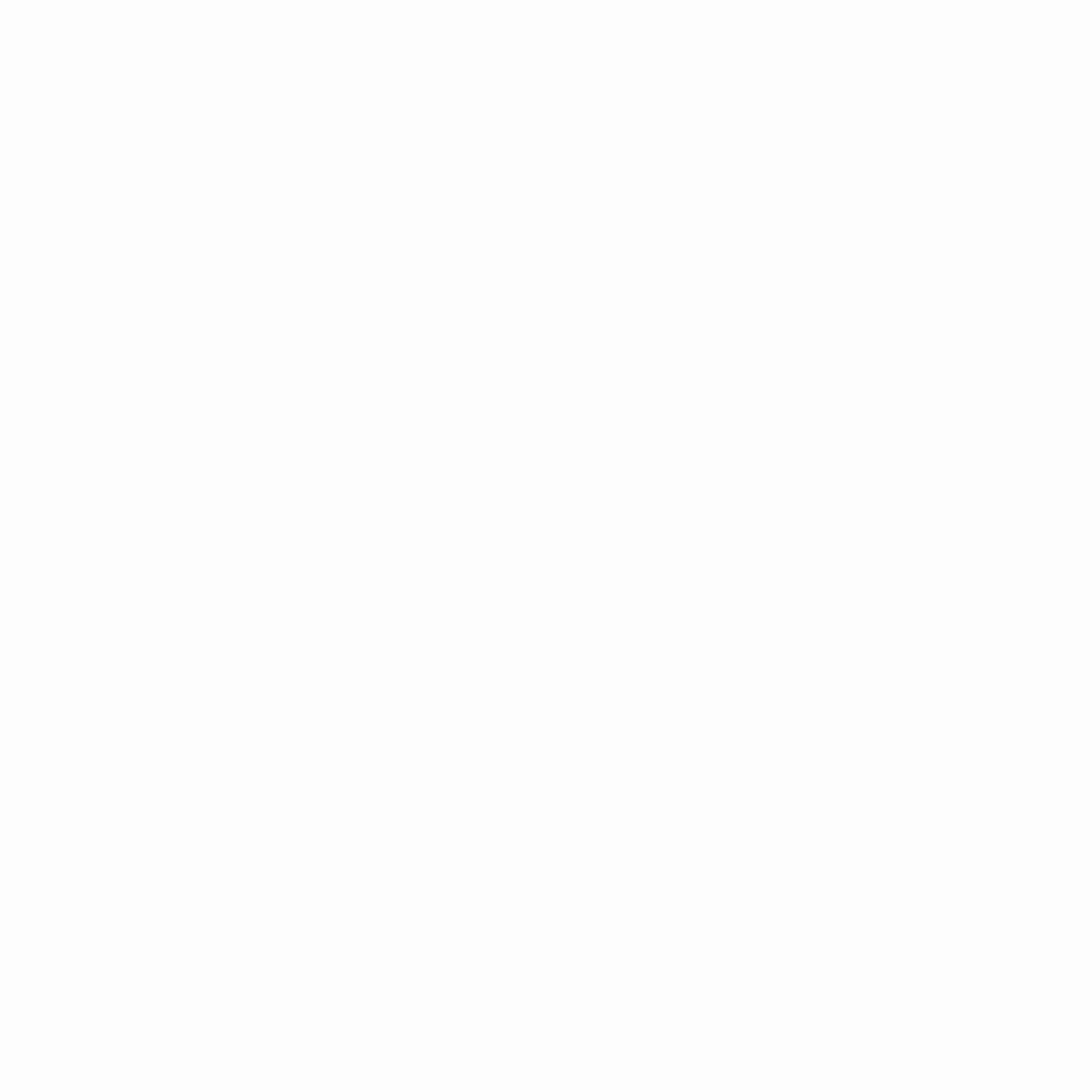
6. Data Cleaning Automation:
To speed up data analytics and obtain reliable insights from big data, many researchers and businesses are seeking techniques to automate data cleaning, which will heavily rely on artificial intelligence and machine learning.
7. Edge Computing:
Edge computing is a technology that allows for data processing not only in the cloud but also in remote locations. This allows businesses to ensure their data is secure and accessible even in areas with limited internet access.
8. Augmented Interfaces:
In future, customers could be able to interact with an AI assistant who can assist them with shopping. They might be purchasing products in virtual reality, hearing an audio description of them, or using an augmented interface.
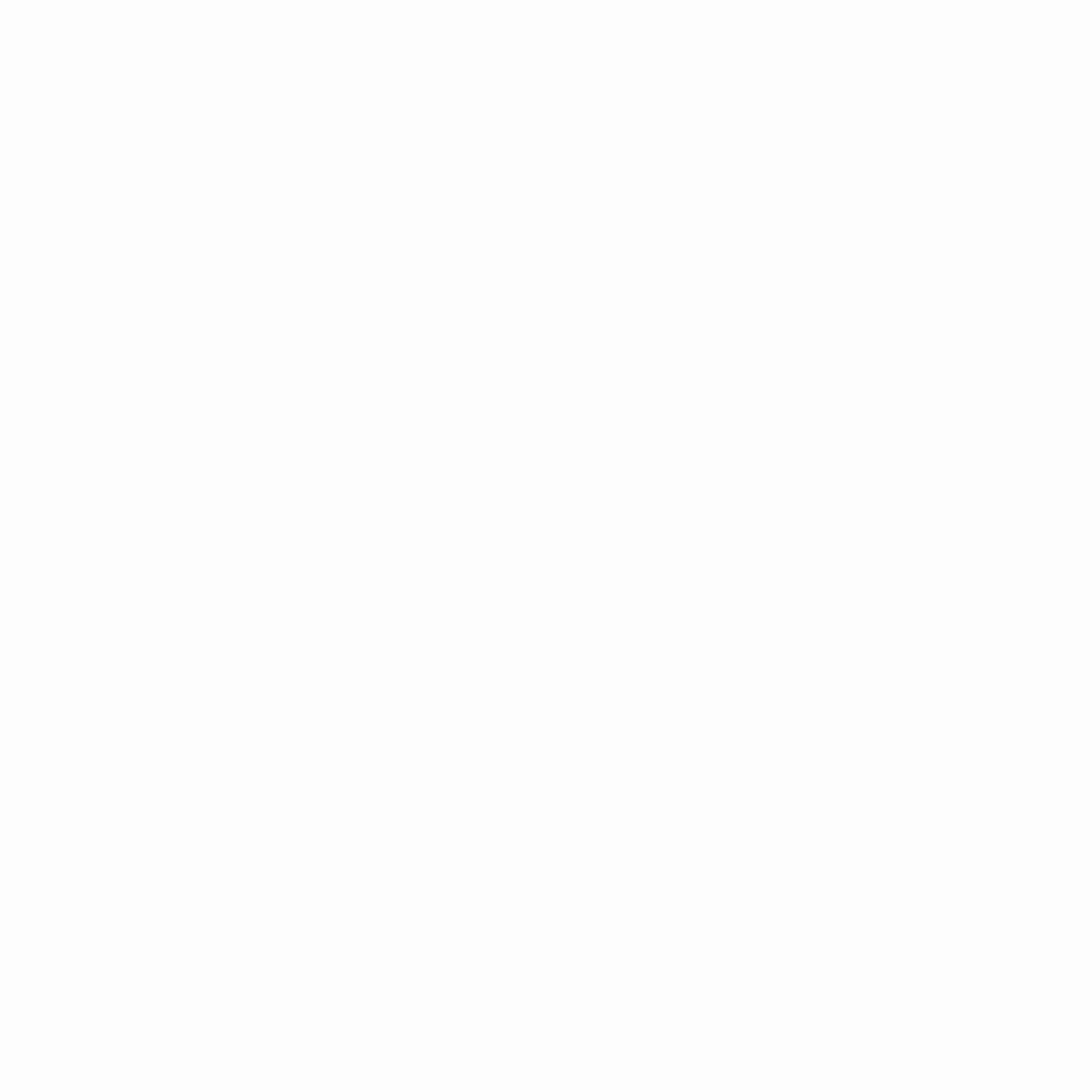
Conclusion
Some of the most important data science trends for 2023 and beyond have been covered in this article. By 2027, it is anticipated that the big data and data analytics industry will surpass $448 billion.
The following graph shows the vital capabilities of industries implementing data science.
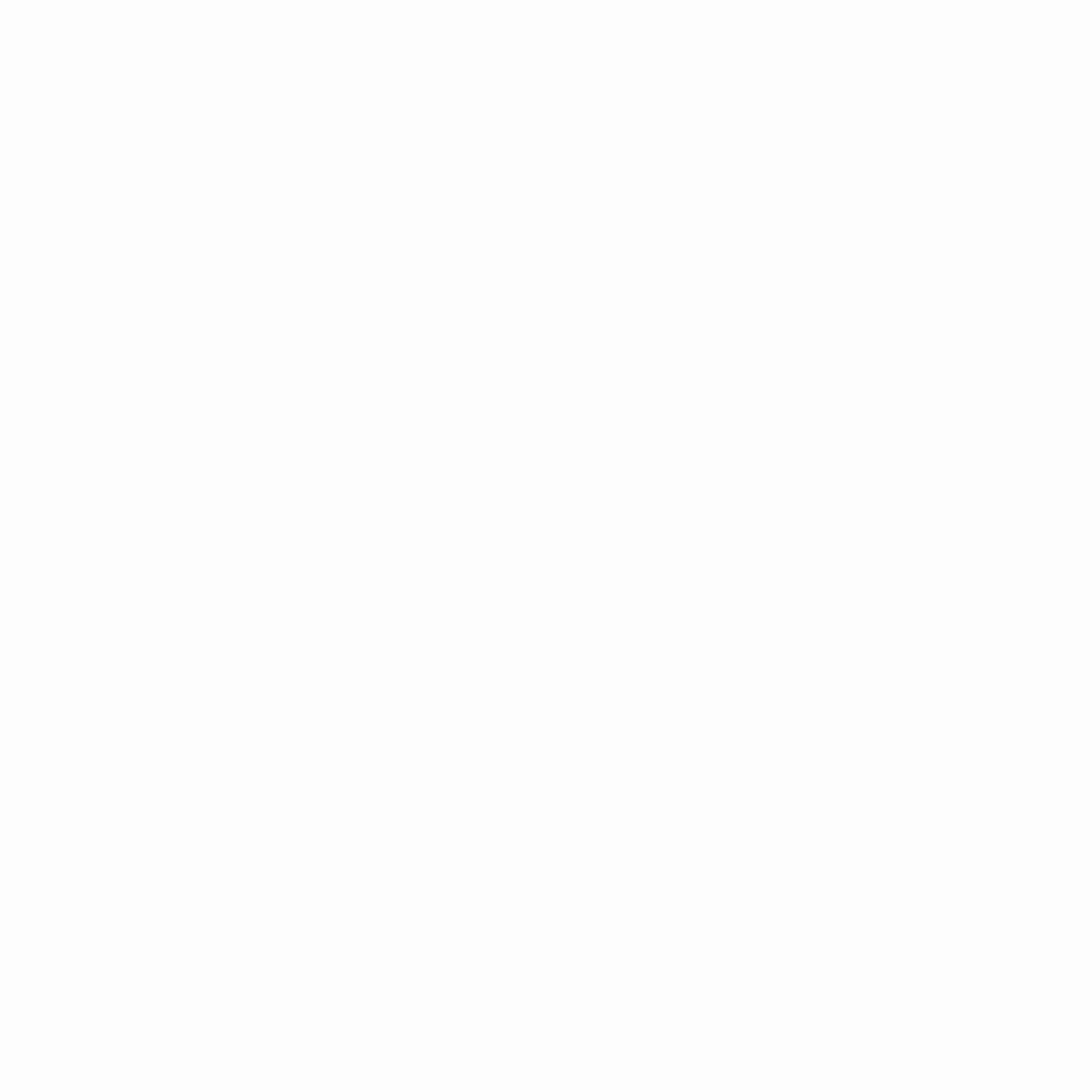
The following graph shows the key areas of big data analytics:
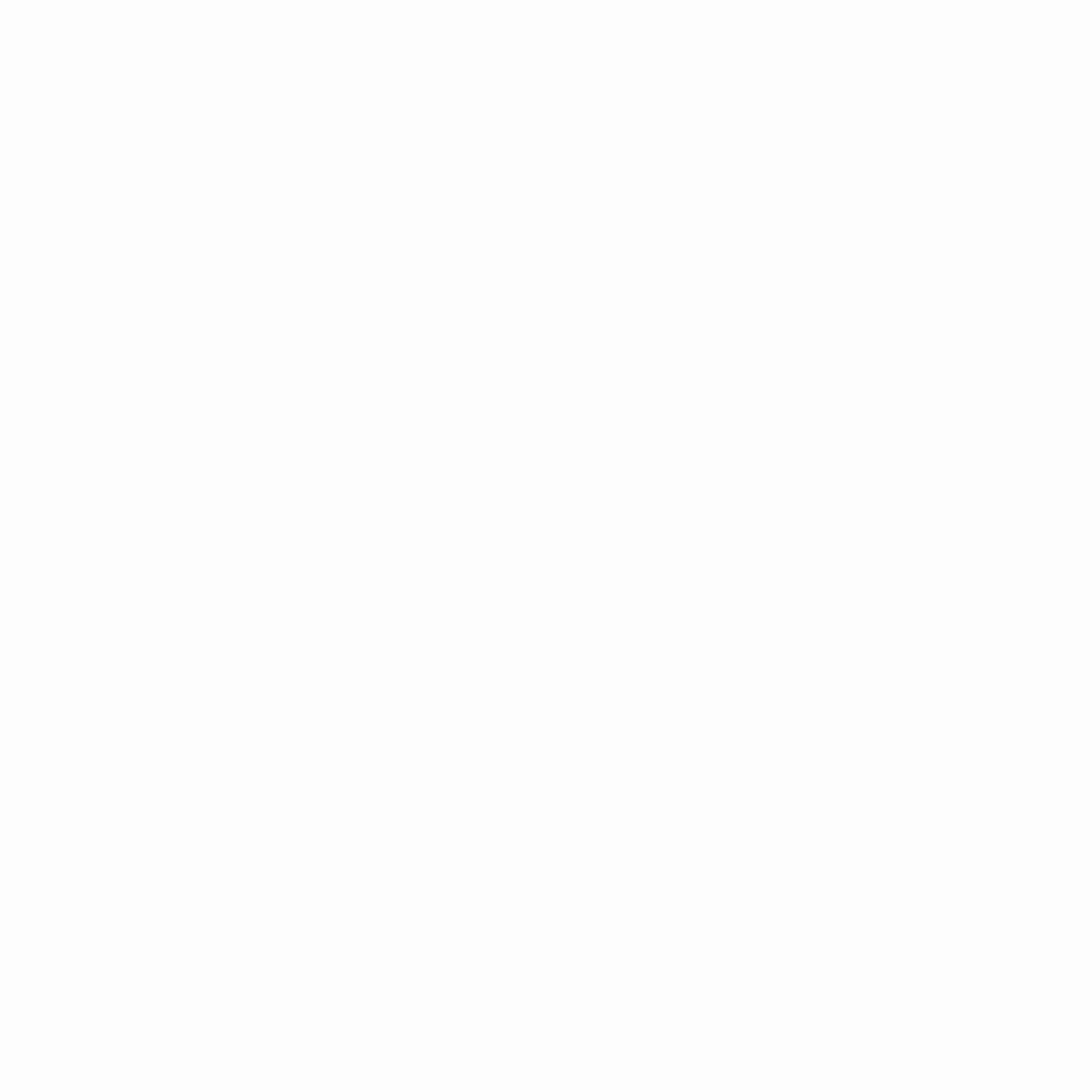
Are you considering a job in data science? Many data science courses are available @ Sambodhi. With experienced instructor-led guidance, practical exposure, mock exams, and top-notch eLearning materials, you’ll be ready to succeed. Our Data Science Career Guide will provide you with information on the trendiest technology, the best employers, the skills needed to launch a successful career in the booming field of data science, and a personalized road map to getting there.